Day 1 :
Keynote Forum
Venkat Lellapalli
Mississippi State University , USA
Keynote: Machine Learning Analysis of Readmission of Patients Diagnosed With Ischemic and Pulmonary Heart Diseases
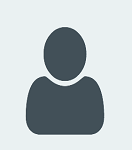
Biography:
Venkat Lellapalli is working on his Ph.D in Industrial and Systems Engineering at the Mississippi State University in USA . He has twenty years of work experience in Healthcare Insurance companies working on Healthcare and wellness projects using Cloud and Machine Learning technologies to improve quality of care for the members
Abstract:
Clinic readmissions are pointers of the nature of administration offered by medical clinics and give a knowledge into the presentation measures on the expense at the clinic. A readmission occasion happens when a patient that has been released from a medical clinic after finding and method is again readmitted to the clinic inside a specific period. The Nationwide Readmissions Database (NRD) is important for a group of information bases and programming instruments created for the Healthcare Cost and Utilization Project (HCUP). For this exploration, the information for the year 2016 from the National Readmission Database (NRD) will be examined and AI models worked to demonstrate the connection among readmission and different variables identified with the patient. The models worked in this examination study will be utilized to facilitate the forecast of medical clinic readmission which is significant in medical care the executives. Ischemic And Pulmonary Heart illnesses are among the basic infections in medical care administrations. The observing of these sicknesses, accordingly, ought to be taken care of with extreme consideration and with prepared experts. Different investigations have demonstrated that readmission of these infections has a higher rate contrasted with non-pneumonic illness, consequently the requirement for basic examination and study in these regions. The perceptions for Ischemic heart ailments and illnesses of aspiratory course (analysis codes I20 to I28) will be utilized for this investigation. Investigation and integrity of model lists, for example, the disarray lattice, AUC list, MSE, and R squared scores and discoveries from the examination will likewise be assessed and revealed considering the model boundaries.
Keynote Forum
Daniel Jeffries
Chief Technical Evangelist, USA
Keynote: Building an AI Red Team to Stop Problems Before They Start
Time : 10:30 - 11:00
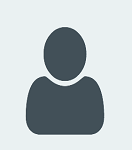
Biography:
Dan Jeffries is Chief Technology Evangelist at Pachyderm. He’s also an author, engineer, futurist, pro blogger and he’s given talks all over the world on AI and cryptographic platforms. He’s spent more than two decades in IT as a consultant and at open source pioneer Red Hat.With more than 50K followers on Medium, his articles have held the number one writer's spot on Medium for Artificial Intelligence, Bitcoin, Cryptocurrency and Economics more than 25 times. His breakout AI tutorial series "Learning AI If You Suck at Math" along with his explosive pieces on cryptocurrency, "Why Everyone Missed the Most Important Invention of the Last 500 Years” and "Why Everyone Missed the Most Mind-Blowing Feature of Cryptocurrency,” are shared hundreds of times daily all over social media and been read by more than 5 million people worldwide.
Abstract:
With algorithms making more and more decisions in our lives, from who gets a job, to who gets hired and fired, and even who goes to jail, it’s more critical than ever to get our intelligent systems talking to us so people can step in when things go wrong. In the coming decade, organizations will face incredible pressure from regulators and the general public and that means every team needs a plan in place to find and fix mistakes fast or risk PR nightmares and financial disasters. I’ll show you how to build an AI Red Team to deal with everything from edge cases to outright AI breakdowns, while getting you ready to embrace the latest breakthroughs in explainable AI tomorrow.
Keynote Forum
Farah Nabillah Abdul Razak
University Putra Malaysia, Malaysia
Keynote: Malaysia License Plate Recognition Based On Deep Learning
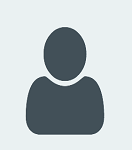
Biography:
Farah Nabillah has completed her Master Degree at the age of 25 years from Universiti Putra Malaysia. She has patented one product based on her degree thesis in 2017 in contribution of detecting freshness of the food using mobile apps. She is an active in volunteering and has a keen interest in AI and education
Abstract:
License plate has been the most important feature in variety of application for example in security and in traffic regulation. Recognizing the license plate research have been conducted for all these application and the data of the license plate can be found either at the toll station or even in parking lot. The important part in detecting vehicle plate is accuracy, speed and the use of limited bandwidth. There are several issues in detecting and recognizing the license plate as the feature of the license plate varies in term of the size of the plate, different standard of license plate and the colour of the license plate. Various technique have been used in detecting the license plate especially in conventional image processing. Conventional image processing involve in thresholding, detection, segmentation and recognition. But there are limitation in conventional image processing. The system use a very complex algorithm and the data have a problem with a illumination and previous work usually keep some parameter constant in order to detect and recognize the license plate. While the conventional image processing lack in the accuracy regarding the illumination, deep learning being used to ease the complexity of the algorithm in the license plate recognition. One of the feature is Deep learning where the data can process al large number of data and the data can be trained and test. Neural network have been chosen as the main feature in detecting and recognizing the license plate
Keynote Forum
Rehan Babar
University of Calgary, Canada
Keynote: Deeper Look: Artificial Intelligence(AI) acceptance in radiology
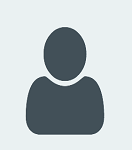
Biography:
Rehan Babar, is currently completing his Bachelor of Commerce Honours at University of Calgary (UofC) with a specialization in Accounting/Finance and Artificial Intelligence (AI). He has represented UofC in some of the most prestigious management competitions in the world (Harvard Case Competition, Inter-collegiate Business Case Competition) and has earned a top-3 spot in 5 global competitions. He is among the first 7 students from the Haskayne School of Business to be selected for the prestigious and rigorous Honours research program and has received multiple awards for his research pertaining to AI in the field of radiology.
Abstract:
Ubiquitous computational power. Faster Data processing. Rapid progress of analytic techniques. We are amid major changes all around us and they are happening at an exponential pace. Artificial Intelligence (AI) – which aims to mimic human cognitive functions – is bringing a paradigm shift to the field of radiology. In the last decade, AI techniques known as deep learning have delivered rapidly improving performance in image recognition, caption generation, and speech recognition. Further implementation of AI in radiology will significantly improve the quality, value, and depth of radiology’s contribution to patient care and revolutionize radiologists’ workflows. However, recent reports of health information technology (IT) show that the acceptance between purchased technologies and clinical work systems is critical in determining intended end users to accept or reject the technology, to use or to misuse it, or to incorporate it into their clinical workflows or work around it. This paper assesses technology implementation frameworks in the context of AI in radiology and employs a widely accepted and validated technology acceptance framework - the Technology Acceptance Model (TAM). The model is built on the premise that when an end-user is introduced to a technology, there are constructs and relationships that influence when and how a user will interact with the technology. In addition, the findings can further inform and provide guidance for policymakers, AI application developers, and business management on the educational needs of radiologists, research and development, and the role of radiologists in moving forward with AI in radiology
Keynote Forum
Shahrzad Yaghtin
University, Tehran, Iran
Keynote: How Machine Learning Can Help the Marketers to Communicate to the Customers More Successfully Through Creating More Relevant Digital Content
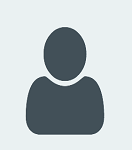
Biography:
Shahrzad Yaghtin is a PhD candidate and a researcher at Business administration department of Central branch of Azad university of Tehran.. With a background of studies focused on Digital content marketing, Innovation marketing, Motivational theories, Data Science and Machine learning and the empirical experience of working at the industry sector, currently she is working on serving data science and machine learning to obtain more reliable results in marketing. In addition to her academic studies, she has the experience of working in the industry sector as a market analyst, marketing expert, and a head of marketing in the industry sector since May 2008.
Abstract:
Digital content marketing is known as a strategic marketing approach focused on producing and distributing valuable, relevant, and consistent digital content to attract target audiences and to retain the customers. Content marketing can yield great results as it is an effective way to reach desired target audiences, also it enriches interactions with customers at every stage of their buyer journey. On the other hand, Machine learning is defined as the study of computer algorithms that allow computer programs to automatically improve through experience. Machine learning relies on working with small to large data-sets by examining and comparing the data to find common patterns and explore nuances. Machine Learning algorithms are characterized by a unique ability to learn system behavior from past data and estimate future responses based on the learned system model. This ability can help the content marketers to identify and provide the more relevant and useful information to the audiences. Therefore, the main purpose of this study is to describe how machine learinng can be used for identifiying more relevant and compelling digital content for improving communicayion with the customers and audiences. For this purpose, data were drawn from the 1156 questionnaires filled by the participants of three conferences and exhibition held in the energy sector. Python in Jupyter environment was used as the main program for data analysis maching learning purposes. In total, 4 main content classes were identified as the most popular and helpful content to communicate to the audiences more successfully.
- Artificial Intelligence
Location: Webinar
Session Introduction
Abir GALLALA
University of Luxembourg, Luxembourg
Title: Augmented-Reality-based digital twin approach for robot manipulation
Biography:
Abir GALLALA has completed her master in 2016 in Artificial Intelligence from the national engineering school of Sousse in Tunisia and a bachelor in industrial computer science from the same school. Currentlz she is pursuing a doctoral degree PhD at the university of Luxembourg in Human-Robot Interaction within the engineering department.
Abstract:
In recent years, robotics research has been facing a significant growing. Industrial and research interests are moving from the development of robots for structured industrial environments to the development of collaborative and autonomous robots operating in hybrid environments. The biggest drawback of the introduction of these cobots (collaborative robots) is that they are not user friendly. The fourth industrial revolution has allowed the introduction of new technologies that enhance the humanrobot interaction such as Augmented Reality (AR). In this poster, we suggest our approach which is an Augmented Reality-based digital twin approach for an easy and user-friendly Human-Robot interaction. The presented system is a marker-based system that allows users to program collaborative robots. This model aims to manioulate a virtual model of the cobot using an AR head-mounted device
Billy Susilo
National Taiwan University of Science and Technology, Taipei, Taiwan
Title: Predicting Microbial Species in a River Based on Physicochemical Properties by Bio-Inspired Metaheuristic Optimized Machine Learning
Biography:
Billy Susilo obtained his B.Eng in civil construction engineering and project management department at Petra Christian University, Surabaya, Indonesia. Afterwards, he received full scholarship from Taiwan government to pursue his master degree at National Taiwan University of Science and Technology, Taipei, Taiwan, and did research collaboration with environmental engineering department of National Taiwan University, Taipei, Taiwan. Besides graduated as best graduated student during his master degree period, he also receive awards as one of the best speaker for his research in 22nd Symposium on Construction Engineering and Management. His research focuses on engineering informatics, optimization on machine learning algorithm and metaheuristic artificial intelligence techniques. Currently, he is a data supervisor senior engineer in one the biggest geotechnical real-time monitoring construction company in Taiwan.
Abstract:
The primary objective of the examination of microbial nature is to comprehend the connection between Earth's microbial network and their capacities in the earth. This paper presents a proof-of-idea exploration to build up a bioclimatic displaying approach that use man-made reasoning procedures to distinguish the microbial species in a stream as a component of physicochemical boundaries. Highlight decrease and determination are both used in the information preprocessing attributable to the scant of accessible information focuses gathered and missing estimations of physicochemical ascribes from a waterway in Southeast China. A bio-roused metaheuristic upgraded machine student, which bolsters the change in accordance with the numerous yield forecast structure, is utilized in bioclimatic demonstrating. The exactness of expectation and pertinence of the model can support microbiologists and scientists in measuring the anticipated microbial species for additional exploratory arranging with negligible use, which is gotten one of the most major issues when confronting emotional changes of natural conditions brought about by an Earth-wide temperature boost. This work exhibits a neoteric approach for expected use in anticipating primer microbial structures in nature.
Jayaraj P B
National Institute of Technology Calicut, India
Title: AI in Computational Drug Discovery
Biography:
He received his Ph.D in Computer Science from National Institute of Technology Calicut, India. His thesis was “GPU based Virtual Screening Techniques for Faster Drug Discovery”. Now he is an assistant professor at the CSE department, NIT Calicut, India. His research interests include Medical-informatics, Computational Drug Design and GPU Computing. He has published many journals as well as conference proceedings. He has attended an International spring school on High Performance Computing (HighPer 2018) at San Sebastian, Spain in April 2018.
Abstract:
Ordinary medication revelation strategies depend essentially in-vitro tries directed with an objective particle and an extremely huge arrangement of little atoms to pick a correct ligand. With the investigation space for the correct ligand being exceptionally huge, this methodology is profoundly tedious and requires high capital for assistance. Virtual screening, a computational method utilized for assessing an enormous gathering of particles to recognize lead atoms, can be utilized for this reason to accelerate the medication revelation measure. Ligand based medication configuration works by building an applied model of the objective protein. Ligand based virtual screening utilizes this model to assess and isolate dynamic particles for an objective protein. A classes of calculation in machine inclining called Classification calculation can be utilized to construct the above model. In this theoretical, 3 distinctive AI ways to deal with settle virtual screening is depicted. The principal strategy uses a proficient virtual screening method utilizing Random Forest (RF) classifier. Second procedure applies SVM classifier for virtual screening. The third technique shows the appropriateness of Self Organizing Map (SOM) as a classifier for screening ligand atoms, which is first of its sort around there according to the writing. The discussion end with looking at the in addition to and short of the three strategies. The GPU parallelisation of these techniques will be additionally clarified in subtleties
Neel Gandhi
Pandit Deendayal Petroleum University, India
Title: Application of Artificial Intelligence in field of urological cancer
Biography:
Neel Gandhi is pursing his Bachelor’s in Information and communication technology having experienced in Artificial Intellgience in Healthcare from Pandit Deendayal Petroleum University.He is associated with IEEE and has done projects in field of artificial intelligence.He has participated in many conferences and interested in research
Abstract:
Artificial intelligence(AI) techniques like artificial neural networks,Bayesian belief networks and neurological fuzzy systems are widely adopted in urology.AI approach are found to be more exploratory as well as accurate in the terms of prediction compared to conventional statistics.The model are complex mathematical based models derived from working of human brain.A detailed study conducted in the field of urology using a technique resulted in finding a new dynamic applications in the field of neurological cancer treatment.The results of machine learning techniques and implementation were focused on handling as well as prediction using artificial neural networks for the purpose of diagnosis,prognosis and treatment of cancer.Different techniques of AI depending on respective characteristics,where found suitable for different tasks.Also,the lack of transparency in neural networks was also overcome using neuro-fuzzy systems.
Biography:
Abstract:
In this presentation we described and implemented Image compression using recurrent neural network, the compression of image method is a type of information compression that will decrease the same amount of image to be transmitted, stored and evaluated, but without losing the information content. Here we are compressing image with one of most type of neural network i.e. Recurrent Neural Network (RNN). The architecture consist of recurrent neural network based encoder, binarizer, and decoder system. Using this reconstructed the image which is having better quality than the original image and along with this here we show the activation function i.e. Sigmoid, ReLU and tanh functions. And also we evaluated PSNR, MSE, CR, BPP and SSIM, MS-SSIM, parameters for comparing original and compressed images. For this we are taken selected images on the Kodak dataset images. And this work is performed by using python 3.6 version tool with some standard packages for AI functions. So this can demonstrates that our Deep learning achieves better generalization.
Biography:
Abstract:
In this presentation we described and implemented Image compression using recurrent neural network, the compression of image method is a type of information compression that will decrease the same amount of image to be transmitted, stored and evaluated, but without losing the information content. Here we are compressing image with one of most type of neural network i.e. Recurrent Neural Network (RNN). The architecture consist of recurrent neural network based encoder, binarizer, and decoder system. Using this reconstructed the image which is having better quality than the original image and along with this here we show the activation function i.e. Sigmoid, ReLU and tanh functions. And also we evaluated PSNR, MSE, CR, BPP and SSIM, MS-SSIM, parameters for comparing original and compressed images. For this we are taken selected images on the Kodak dataset images. And this work is performed by using python 3.6 version tool with some standard packages for AI functions. So this can demonstrates that our Deep learning achieves better generalization.